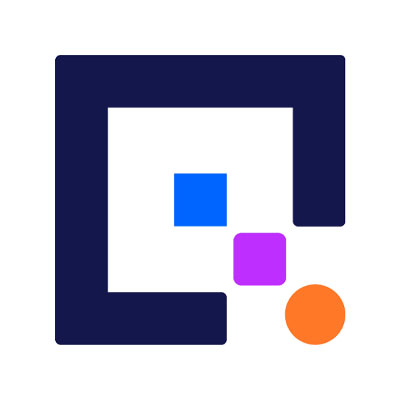
Quix for InfluxDB
免费使用此InfluxDB集成为什么使用InfluxDB的Quix?
InfluxDB的Quix允许您构建实时数据管道,在Python中进行数据预处理并触发操作。如果您已经了解Python,它是对Kapacitor和Flux的完美替代,因为它可以执行相同的一些常见任务,例如数据集成、丰富、降采样和警报。您可以使用Quix在任何需要在外部系统中检测事件并触发操作的场景中,例如异常检测和预测性维护。
Quix由Quix Streams和Quix Cloud组成。Quix Streams是一个开源Python客户端库,具有流式DataFrame和状态操作,如窗口函数和自定义聚合。它为Apache Kafka提供了高级抽象,使Python开发者能够以弹性和持久性保证与流式数据进行工作。作为开源软件,您的业务逻辑没有供应商锁定。Quix Streams可在安装了Python 3.8+的任何地方运行。
Quix Cloud是一个用于在流式ETL管道中运行Quix Streams任务的完全托管平台。您可以使用云IDE和可视DAG编辑器构建管道,并一键部署。Quix Cloud提供托管Apache Kafka、Kubernetes、监控和CI/CD。您可以使用它作为无服务器平台,或将其部署到本地基础设施以构建混合边缘到云数据平台。
如何使用此集成
要使用此集成,您需要在某处运行Apache Kafka——Quix使用Kafka作为其数据骨干来存储消息、警报和中间计算/聚合。您可以将自己的Kafka集群带到Quix Cloud上,或使用Quix Cloud上托管的Kafka。
您可以在Quix网站上免费试用帐户。
一旦您有了帐户,请创建一个项目,创建一个初始环境,然后创建一个应用程序。转到代码示例,并定位到InfluxDB 3.0源连接器。
定义以下环境变量
- 输出:将接收流数据的输出Kafka主题名称。如果该主题不存在,则在第一次运行时自动创建。(默认值:influxdb,必需:是)
- task_interval:运行查询的间隔。必须使用InfluxDB记法;1s、1m、1h、1d、1w、1mo、1y(默认值:5m,必需:是)
- INFLUXDB_HOST:InfluxDB实例的主机地址。(默认值:eu-central-1-1.aws.cloud2.influxdata.com,必需:是)
- NFLUXDB_TOKEN:访问InfluxDB的认证令牌。(默认值:
TOKEN
,必需:是) - INFLUXDB_ORG:InfluxDB中的组织名称。(默认值:
ORG
,必需:否) - INFLUXDB_DATABASE:存储数据的InfluxDB中的数据库名称。(默认值:
DATABASE
,必需:是) - INFLUXDB_MEASUREMENT_NAME:从其中读取数据的InfluxDB度量。如果不指定,则使用输出主题的名称。(必需:否)
以下代码在Quix IDE中运行,您将在创建您的第一个应用程序后看到它(您也可以通过从代码使用Quix示例库创建应用程序来在本地工作)。
# Import utility modules
import os
import random
import json
import logging
from time import sleep
# Import vendor-specific libraries
from quixstreams import Application
import influxdb_client_3 as InfluxDBClient3
# Initialize logging
logging.basicConfig(level=logging.INFO)
logger = logging.getLogger(__name__)
# Create a Quix Application
app = Application(consumer_group="influxdb_sample", auto_create_topics=True)
# Define the topic using the "output" environment variable, along with how its
# data should be serialized
topic = app.topic(
name=os.environ["output"],
key_serializer="string",
value_serializer="json"
)
influxdb3_client = InfluxDBClient3.InfluxDBClient3(
token=os.environ["INFLUXDB_TOKEN"],
host=os.environ["INFLUXDB_HOST"],
org=os.environ["INFLUXDB_ORG"],
database=os.environ["INFLUXDB_DATABASE"]
)
measurement_name = os.getenv("INFLUXDB_MEASUREMENT_NAME", os.environ["output"])
interval = os.getenv("task_interval", "5m")
# Global variable to control the main loop's execution
run = True
# InfluxDB interval-to-seconds conversion dictionary
UNIT_SECONDS = {
"s": 1,
"m": 60,
"h": 3600,
"d": 86400,
"w": 604800,
"y": 31536000,
}
# Helper function to convert time intervals (like 1h, 2m) into seconds for easier processing.
# This function is useful for determining the frequency of certain operations.
def interval_to_seconds(interval: str) -> int:
try:
return int(interval[:-1]) * UNIT_SECONDS[interval[-1]]
except ValueError as e:
if "invalid literal" in str(e):
raise ValueError(
"interval format is {int}{unit} i.e. '10h'; "
f"valid units: {list(UNIT_SECONDS.keys())}")
except KeyError:
raise ValueError(
f"Unknown interval unit: {interval[-1]}; "
f"valid units: {list(UNIT_SECONDS.keys())}")
interval_seconds = interval_to_seconds(interval)
# Function to fetch data from InfluxDB and send it to Quix
# It runs in a continuous loop, periodically fetching data based on the interval.
def get_data():
# Run in a loop until the main thread is terminated
while run:
try:
query_definition = f'SELECT * FROM "{measurement_name}" WHERE time >= now() - {interval}'
logger.info(f"Sending query {query_definition}")
# Query InfluxDB 3.0 using influxql or sql
table = influxdb3_client.query(
query=query_definition,
mode="pandas",
language="influxql"
)
table = table.drop(columns=["iox::measurement"])
# If there are rows to write to the stream at this time
if not table.empty:
# Convert to JSON for JSON-to-bytes serializer
json_result = table.to_json(orient='records', date_format='iso')
yield json_result
logger.info("query success")
else:
logger.info("No new data to publish.")
# Wait for the next interval
sleep(interval_seconds)
except Exception as e:
logger.error(f"query failed; error: {e}")
sleep(1)
def main():
"""
Read data from the Query and publish it to Kafka
"""
# Create a pre-configured Producer object.
# Producer is already setup to use Quix brokers.
# It will also ensure that the topics exist before producing to them if
# Application is initialized with "auto_create_topics=True".
with app.get_producer() as producer:
for res in get_data():
# Parse the JSON string into a Python object
records = json.loads(res)
for index, obj in enumerate(records):
# Generate a unique message_key for each row
message_key = f"INFLUX_DATA_{str(random.randint(1, 100)).zfill(3)}_{index}"
logger.info(f"Produced message with key:{message_key}, value:{obj}")
# Serialize data for kafka producing
serialized = topic.serialize(key=message_key, value=obj)
# publish the data to the topic
producer.produce(
topic=topic.name,
key=serialized.key,
value=serialized.value,
)
if __name__ == "__main__":
try:
main()
except KeyboardInterrupt:
logger.info("Stop requested...")
run = False
finally:
logger.info("Application has been stopped")
有关如何运行和部署应用程序的更多详细信息,请参阅Quix文档。
一旦数据在Kafka主题中可用,您就可以使用另一个服务来消费和处理数据(您可以将常见的InfluxDB任务,如下采样迁移过来)。当您准备好将数据写回InfluxDB时,使用相应的输出InfluxDB 3.0 Sink连接器。
为了获得灵感,请参阅Quix模板库,其中包含可运行的参考用例/数据管道和完整源代码。您可能还对在InfluxDB v2和v3之间同步数据以及使用InfluxDB进行预测性维护感兴趣。
注意:此处涵盖的连接器适用于InfluxDB 3.0,但在Quix Samples GitHub存储库中还有可用的输入InfluxDB 2.0 Source连接器。